|
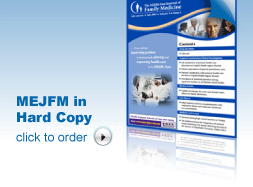 |
............................................................. |
October 2017 -
Volume 15, Issue 8
|
 |
View
this issue in pdf formnat - the issue
has been split into two files for downloading
due to its large size: FULLpdf
(12 MB)
Part
1 &
Part
2
|
|
........................................................ |
From
the Editor |

|
Editorial
A. Abyad (Chief Editor) |
........................................................
|
Original Contribution/Clinical Investigation
Immunity
level to diphtheria in beta thalassemia patients
DOI: 10.5742/MEWFM.2017.93048
[pdf
version]
Abdolreza Sotoodeh Jahromi, Karamatollah Rahmanian,
Abdolali Sapidkar, Hassan Zabetian, Alireza
Yusefi, Farshid Kafilzadeh, Mohammad Kargar,
Marzieh Jamalidoust,
Abdolhossein Madani
Genetic
Variants of Toll Like Receptor-4 in Patients
with Premature Coronary Artery Disease, South
of Iran
DOI: 10.5742/MEWFM.2017.93049
[pdf
version]
Saeideh Erfanian, Mohammad Shojaei, Fatemeh
Mehdizadeh, Abdolreza Sotoodeh Jahromi, Abdolhossein
Madani, Mohammad Hojjat-Farsangi
Comparison
of postoperative bleeding in patients undergoing
coronary artery bypass surgery in two groups
taking aspirin and aspirin plus CLS clopidogrel
DOI: 10.5742/MEWFM.2017.93050
[pdf
version]
Ali Pooria, Hassan Teimouri, Mostafa Cheraghi,
Babak Baharvand Ahmadi, Mehrdad Namdari, Reza
Alipoor
Comparison
of lower uterine segment thickness among nulliparous
pregnant women without uterine scar and pregnant
women with previous cesarean section: ultrasound
study
DOI: 10.5742/MEWFM.2017.93051
[pdf version]
Taravat Fakheri, Irandokht Alimohammadi, Nazanin
Farshchian, Maryam Hematti,
Anisodowleh Nankali, Farahnaz Keshavarzi, Soheil
Saeidiborojeni
Effect
of Environmental and Behavioral Interventions
on Physiological and Behavioral Responses of
Premature Neonates Candidates Admitted for Intravenous
Catheter Insertion in Neonatal Intensive Care
Units
DOI: 10.5742/MEWFM.2017.93052
[pdf
version]
Shohreh Taheri, Maryam Marofi, Anahita Masoumpoor,
Malihe Nasiri
Effect
of 8 weeks Rhythmic aerobic exercise on serum
Resistin and body mass index of overweight and
obese women
DOI: 10.5742/MEWFM.2017.93053
[pdf
version]
Khadijeh Molaei, Ahmad Shahdadi, Reza Delavar
Study
of changes in leptin and body mass composition
with overweight and obesity following 8 weeks
of Aerobic exercise
DOI: 10.5742/MEWFM.2017.93054
[pdf
version]
Khadijeh Molaei, Abbas Salehikia
A reassessment
of factor structure of the Short Form Health
Survey (SF-36): A comparative approach
DOI: 10.5742/MEWFM.2017.93088
[pdf version]
Vida Alizad, Manouchehr Azkhosh, Ali Asgari,
Karyn Gonano
Population and Community Studies
Evaluation
of seizures in pregnant women in Kerman - Iran
DOI: 10.5742/MEWFM.2017.93056
[pdf
version]
Hossein Ali Ebrahimi, Elahe Arabpour, Kaveh
Shafeie, Narges Khanjani
Studying
the relation of quality work life with socio-economic
status and general health among the employees
of Tehran University of Medical Sciences (TUMS)
in 2015
DOI: 10.5742/MEWFM.2017.93057
[pdf version]
Hossein Dargahi, Samereh Yaghobian, Seyedeh
Hoda Mousavi, Majid Shekari Darbandi, Soheil
Mokhtari, Mohsen Mohammadi, Seyede Fateme Hosseini
Factors
that encourage early marriage and motherhood
from the perspective of Iranian adolescent mothers:
a qualitative study
DOI: 10.5742/MEWFM.2017.93058
[pdf
version]
Maasoumeh Mangeli, Masoud Rayyani, Mohammad
Ali Cheraghi, Batool Tirgari
The
Effectiveness of Cognitive-Existential Group
Therapy on Reducing Existential Anxiety in the
Elderly
DOI: 10.5742/MEWFM.2017.93059
[pdf
version]
Somayeh Barekati, Bahman Bahmani, Maede Naghiyaaee,
Mahgam Afrasiabi, Roya Marsa
Post-mortem
Distribution of Morphine in Cadavers Body Fluids
DOI: 10.5742/MEWFM.2017.93060
[pdf
version]
Ramin Elmi, Mitra Akbari, Jaber Gharehdaghi,
Ardeshir Sheikhazadi, Saeed Padidar, Shirin
Elmi
Application
of Social Networks to Support Students' Language
Learning Skills in Blended Approach
DOI: 10.5742/MEWFM.2017.93061
[pdf
version]
Fatemeh Jafarkhani, Zahra Jamebozorg, Maryam
Brahman
The
Relationship between Chronic Pain and Obesity:
The Mediating Role of Anxiety
DOI: 10.5742/MEWFM.2017.93062
[pdf
version]
Leila Shateri, Hamid Shamsipour, Zahra Hoshyari,
Elnaz Mousavi, Leila Saleck, Faezeh Ojagh
Implementation
status of moral codes among nurses
DOI: 10.5742/MEWFM.2017.93063
[pdf
version]
Maryam Ban, Hojat Zareh Houshyari Khah, Marzieh
Ghassemi, Sajedeh Mousaviasl, Mohammad Khavasi,
Narjes Asadi, Mohammad Amin Harizavi, Saeedeh
Elhami
The comparison
of quality of life, self-efficacy and resiliency
in infertile and fertile women
DOI: 10.5742/MEWFM.2017.93064
[pdf version]
Mahya Shamsi Sani, Mohammadreza Tamannaeifar
Brain MRI Findings in Children (2-4 years old)
with Autism
DOI: 10.5742/MEWFM.2017.93055
[pdf
version]
Mohammad Hasan Mohammadi, Farah Ashraf Zadeh,
Javad Akhondian, Maryam Hojjati,
Mehdi Momennezhad
Reviews
TECTA gene function and hearing: a review
DOI: 10.5742/MEWFM.2017.93065
[pdf version]
Morteza Hashemzadeh-Chaleshtori, Fahimeh Moradi,
Raziyeh Karami-Eshkaftaki,
Samira Asgharzade
Mandibular
canal & its incisive branch: A CBCT study
DOI: 10.5742/MEWFM.2017.93066
[pdf
version]
Sina Haghanifar, Ehsan Moudi, Ali Bijani, Somayyehsadat
Lavasani, Ahmadreza Lameh
The
role of Astronomy education in daily life
DOI: 10.5742/MEWFM.2017.93067
[pdf
version]
Ashrafoalsadat Shekarbaghani
Human brain
functional connectivity in resting-state fMRI
data across the range of weeks
DOI: 10.5742/MEWFM.2017.93068
[pdf version]
Nasrin Borumandnia, Hamid Alavi Majd, Farid
Zayeri, Ahmad Reza Baghestani,
Mohammad Tabatabaee, Fariborz Faegh
International Health Affairs
A
brief review of the components of national strategies
for suicide prevention suggested by the World
Health Organization
DOI: 10.5742/MEWFM.2017.93069
[pdf
version]
Mohsen Rezaeian
Education and Training
Evaluating
the Process of Recruiting Faculty Members in
Universities and Higher Education and Research
Institutes Affiliated to Ministry of Health
and Medical Education in Iran
DOI: 10.5742/MEWFM.2017.93070
[pdf
version]
Abdolreza Gilavand
Comparison
of spiritual well-being and social health among
the students attending group and individual
religious rites
DOI: 10.5742/MEWFM.2017.93071
[pdf
version]
Masoud Nikfarjam, Saeid Heidari-Soureshjani,
Abolfazl Khoshdel, Parisa Asmand, Forouzan Ganji
A
Comparative Study of Motivation for Major Choices
between Nursing and Midwifery Students at Bushehr
University of Medical Sciences
DOI: 10.5742/MEWFM.2017.93072
[pdf
version]
Farzaneh Norouzi, Shahnaz Pouladi, Razieh Bagherzadeh
Clinical Research and Methods
Barriers
to the management of ventilator-associated pneumonia:
A qualitative study of critical care nurses'
experiences
DOI: 10.5742/MEWFM.2017.93073
[pdf version]
Fereshteh Rashnou, Tahereh Toulabi, Shirin Hasanvand,
Mohammad Javad Tarrahi
Clinical
Risk Index for Neonates II score for the prediction
of mortality risk in premature neonates with
very low birth weight
DOI: 10.5742/MEWFM.2017.93074
[pdf
version]
Azadeh Jafrasteh, Parastoo Baharvand, Fatemeh
Karami
Effect
of pre-colporrhaphic physiotherapy on the outcomes
of women with pelvic organ prolapse
DOI: 10.5742/MEWFM.2017.93075
[pdf
version]
Mahnaz Yavangi, Tahereh Mahmoodvand, Saeid Heidari-Soureshjani
The
effect of Hypertonic Dextrose injection on the
control of pains associated with knee osteoarthritis
DOI: 10.5742/MEWFM.2017.93076
[pdf
version]
Mahshid Ghasemi, Faranak Behnaz, Mohammadreza
Minator Sajjadi, Reza Zandi,
Masoud Hashemi
Evaluation
of Psycho-Social Factors Influential on Emotional
Divorce among Attendants to Social Emergency
Services
DOI: 10.5742/MEWFM.2017.93077
[pdf
version]
Farangis Soltanian
Models and Systems of Health Care
Organizational
Justice and Trust Perceptions: A Comparison
of Nurses in public and private hospitals
DOI: 10.5742/MEWFM.2017.93078
[pdf
version]
Mahboobeh Rajabi, Zahra Esmaeli Abdar, Leila
Agoush
Case series and Case reports
Evaluation
of Blood Levels of Leptin Hormone Before and
After the Treatment with Metformin
DOI: 10.5742/MEWFM.2017.93079
[pdf
version]
Elham Jafarpour
Etiology,
Epidemiologic Characteristics and Clinical Pattern
of Children with Febrile Convulsion Admitted
to Hospitals of Germi and Parsabad towns in
2016
DOI: 10.5742/MEWFM.2017.93080
[pdf
version]
Mehri SeyedJavadi, Roghayeh Naseri, Shohreh
Moshfeghi, Irandokht Allahyari, Vahid Izadi,
Raheleh Mohammadi,
Faculty development
The
comparison of the effect of two different teaching
methods of role-playing and video feedback on
learning Cardiopulmonary Resuscitation (CPR)
DOI: 10.5742/MEWFM.2017.93081
[pdf
version]
Yasamin Hacham Bachari, Leila Fahkarzadeh, Abdol
Ali Shariati
Office based family medicine
Effectiveness
of Group Counseling With Acceptance and Commitment
Therapy Approach on Couples' Marital Adjustment
DOI: 10.5742/MEWFM.2017.93082
[pdf
version]
Arash Ziapour, Fatmeh Mahmoodi, Fatemeh Dehghan,
Seyed Mehdi Hoseini Mehdi Abadi,
Edris Azami, Mohsen Rezaei
|
Chief
Editor -
Abdulrazak
Abyad
MD, MPH, MBA, AGSF, AFCHSE
.........................................................
Editorial
Office -
Abyad Medical Center & Middle East Longevity
Institute
Azmi Street, Abdo Center,
PO BOX 618
Tripoli, Lebanon
Phone: (961) 6-443684
Fax: (961) 6-443685
Email:
aabyad@cyberia.net.lb
.........................................................
Publisher
-
Lesley
Pocock
medi+WORLD International
11 Colston Avenue,
Sherbrooke 3789
AUSTRALIA
Phone: +61 (3) 9005 9847
Fax: +61 (3) 9012 5857
Email:
lesleypocock@mediworld.com.au
.........................................................
Editorial
Enquiries -
abyad@cyberia.net.lb
.........................................................
Advertising
Enquiries -
lesleypocock@mediworld.com.au
.........................................................
While all
efforts have been made to ensure the accuracy
of the information in this journal, opinions
expressed are those of the authors and do not
necessarily reflect the views of The Publishers,
Editor or the Editorial Board. The publishers,
Editor and Editorial Board cannot be held responsible
for errors or any consequences arising from
the use of information contained in this journal;
or the views and opinions expressed. Publication
of any advertisements does not constitute any
endorsement by the Publishers and Editors of
the product advertised.
The contents
of this journal are copyright. Apart from any
fair dealing for purposes of private study,
research, criticism or review, as permitted
under the Australian Copyright Act, no part
of this program may be reproduced without the
permission of the publisher.
|
|
|
October 2017 -
Volume 15, Issue 8 |
|
Human brain functional
connectivity in resting-state fMRI data across
the range of weeks
Nasrin Borumandnia (1)
Hamid Alavi Majd (1)
Farid Zayeri (1)
Ahmad Reza Baghestani (1)
Mohammad Tabatabaee (2)
Fariborz Faeghi (3)
(1) Biostatistics
Department, Faculty of Paramedical Sciences,
Shahid Beheshti University of Medical Sciences,
Tehran, Iran.
(2) Medical Informatics Department, Faculty
of Paramedical Sciences, Shahid Beheshti University
of Medical Sciences, Tehran, Iran.
(3) Radiology Technology Department, Faculty
of Paramedical Sciences, Shahid Beheshti University
of Medical Sciences, Tehran, Iran.
Correspondence:
:Hamid Alavi Majd
Biostatistics Department,
Faculty of Paramedical Sciences,
Shahid Beheshti University of Medical Sciences,
Tehran, Iran
Email: alavimajd@gmail.com
Abstract
Around
15 years after the invention of fMRI,
Functional Connectivity, FC, in the human
brain has emerged as a major issue in
neuroimaging studies. The reason is that
the brain regions are a complex network
of functional communication that plays
a key role in cognitive processes. FC
is defined as the temporal correlation
of neural activation across different
regions of the brain. Functional connectivity
of a single subject seems to be affected
by their situation. The results of the
other studies demonstrate that healthy
brain function shows rich dynamics over
the course of time. So it may be a good
idea to investigate the FC network as
a summary of repeatedly measured fMRI
sessions over more than one time point.
Few studies have been done on the coordination
of neural activity over longitudinal sessions.
This study evaluates the FC cross-subject
averaging of a single individual repeatedly
measured over 16 weeks using the My Connectome
study. Resting state fMRI data were acquired
in some longitudinal sessions. A variance
based linear model, proposed by Fiecas
et al. was employed to conduct statistical
inference on FC patterns of a single human
averaged across time. This model estimates
the autocorrelation structure in a session-specific
manner, and estimates the variance due
to the heterogeneity across sessions.
Key words:
Resting State fMRI, Functional Connectivity,
Variance Components Mode
|
Resting state fMRI, called rs-fMRI, is a method
of functional magnetic resonance imaging, of
fMRI, which is used to evaluate brain activation
that occurs when a subject is not performing
a typical task (1). Brain activity is observed
through changes in Blood Oxygen Level Dependent,
BOLD, signals in the brains voxels. Brain
activity is present even in the absence of an
external task, so BOLD signals will change in
brain regions during a resting state.
One of the important tasks, which has received
interest in recent years, is detecting of brain
areas connectivity. In general, connectivity
investigates how brain regions interact with
each other (2). Functional connectivity, FC,
identifies regions of the brain showing similar
temporal characteristics. In other words, it
can be defined as the temporal correlation between
spatially different brain regions. Usually,
functional connectivity is determined during
the resting state fMRI and it is analyzed in
terms of correlation and spatial clustering
based on temporal similarities in BOLD signals
(3).
In fact, the statistical inference for functional
connectivity are based on statistical measures
of dependency among brain areas. In this way,
some methods are based on temporal correlations
between Regions of Interest, ROIs, or between
a seed region and other voxels throughout
the brain (4). The other common approaches are
clustering and multivariate statistical methods.
Clustering approaches partition the brain into
regions that exhibit similar BOLD signal characteristics
over time. Multivariate methods are used for
dimension reduction, such as Principal Components
Analysis, PCA, and Independent Components Analysis,
ICA. These methods determine spatial patterns
that include most of the variability in the
BOLD time-series (57). In addition, there
are some specific approaches such as Graphical
Lasso, GLasso, and Bayesian non-parametric models
(1,8,9).
It is a fact that functional connectivity changes
over time (10). Therefore, it may be a good
idea that the functional connectivity is considered
during some sessions. So we investigated the
FC network as a summary of repeatedly measured
fMRI sessions over more than one time point,
by averaging of a single individual repeatedly
measured over 16 weeks using the My Connectome
study (11).
Recently, Fiecas et al. have presented a variance-based
method for comparing the FC networks between
a group of patients and a group of healthy controls
in a multi-subject resting-state fMRI data set
(12). They introduced a variance components
framework for modeling the FC networks that
accounts for the autocorrelation inherent in
the ROI time series of each subject and for
subject heterogeneity. We have used their approach,
by replacing the subjects with repeated sessions.
Therefore, we have applied their model and estimated
a functional connectivity pattern for a single
subject based on repeated resting state fMRI
acquired across some weeks.
1. Statistical Inference
To perform statistical inference on the FC network,
we used the proposed model by Fiecas et al.
(12). We applied their approach by considering
sessions instead of subjects. In this way, the
model accounts for the temporal correlation
in the time series within the subject, the covariance
between the different pairs of ROIs within the
subject, and the variability due to the sampling
across sessions. Suppose data include p ROIs,
across N sessions. So the number of paired ROIs
are q=p(p-1)/2 for each
session. Then the model is in the following
form
(1)
Where the Y=(r_11,
,r_q1,r_12,
,r_q2,
,r_1N,
,r_qN)
is the vector of sample correlation coefficients
stacked vertically across the sessions. The
and are vectors with dimension Nq*1.
The q elements of vector are the parameters
of interest that capture the true FC. The model
has two error terms. The first one is used to
model variance and covariance related to the
temporal autocorrelation in the ROI time series
within the subject. The second one represents
the amount of variability that can be attributed
due to sampling across weeks.
Parameters estimated were obtained using the
approach detailed in Fiecas et al. (12).
2. Database
We used data from the My Connectome study that
consists of 89 sessions of resting state fMRI
data on a single healthy human. The My Connectome
project has characterized how the brain of one
person changes over the course of more than
one year. This data was obtained from the Open
fMRI database. Its accession number is ds000031.
We considered resting state fMRI data repeatedly
measured over 16 weeks. The rs-fMRI acquiring
was performed in 89 sessions throughout the
data collection period in the production phase,
using a multi-band EPI sequence (TR=1.16ms,
TE=30ms), voxel size=2.4*2.4*2mm. Starting with
session 27 (December 12 2012). The size of images
was 2.4*2.4*2.4. Image pre-processing was carried
out with the FMRIB Software Library, FSL software
(https://fsl.fmrib.ox.ac.uk/fsl/fslwiki) (13).
Resting state processing included motion correction
(14), removal of non-brain structures (15),
spatial smoothing (5 mm FWHM), and high-pass
temporal filtering.
The goal of this study was to provide comprehensive
patterns of FC cross-session averaging. We specify
the ROIs based on Broodman atlas including 42
ROI. Time courses for each ROI were obtained
by averaging across all voxels within the ROI.
Three ROIs were discarded from the analysis,
because their time series had not been reached.
Then we considered all the pairwise correlations
between the ROI time series, 741 pairwise.
An
individual
subject
FC
was
generated
using
data
from
16
resting
state
sessions
for
39
ROIs
following
the
procedure
described
in
the
previous
section.
A
list
of
the
39
ROIs
with
their
abbreviations
is
presented
in
Table
1.
In
Figure
1,
we
show
the
beta
parameters
that
capture
true
FC
estimated
based
on
longitudinal
sessions,
and
also
the
beta
parameters
for
the
FC
networks
in
16
sessions,
individually.
The
overall
betas
have
more
variance
related
to
the
betas
for
each
of
the
16
sessions.
In
addition,
Figure
1
includes
the
correlations
between
ROIs
averaged
over
the
longitudinal
sessions
and
the
correlations
among
ROI
for
all
16
sessions.
The
image
shows
that
the
correlations
between
paired
ROIs
have
different
variation
during
the
sessions.
Click
here
for
Table
1:
A
list
of
the
ROIs
and
their
numbers
in
analyzing
process
Figure
1:
Up:
The
estimated
beta
over
sessions;
Down:
The
correlations
between
ROI
pairs
over
sessions
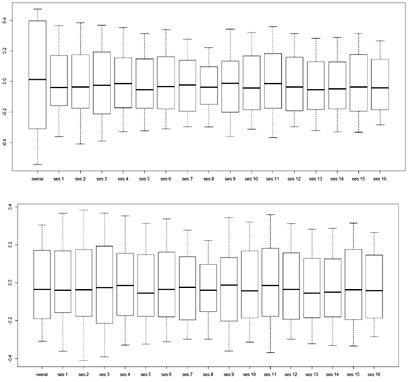
Also,
we
have
shown
the
beta
parameters
that
capture
true
FC
estimate
based
on
longitudinal
session
and
the
beta
parameters
for
session
1
and
vice
versa
in
Figure
2,
in
the
upper
triangle
and
lower
triangle,
respectively.
In
this
image,
we
can
see
the
difference
between
the
estimated
betas
related
to
each
of
the
ROI
pairs
in
detail.
FC
networks
for
sessions
numbers
1,
8
and
16
also
drawn
vice
versa
in
the
overall
FC
network
in
Figure
2.
These
Results
show
that
the
FC
networks
are
not
static
across
the
sessions.
Click
here
for
Figure
2.
Upper
triangle:
the
estimated
beta
totally.
Lower
triangle:
the
estimated
beta
for
Session
1
Click
here
for
Figure
3.
(a)
The
estimated
betas
for
Session
1;
(b)
The
estimated
betas
for
Session
8;
(c)
The
estimated
betas
for
Session
16
The
human
brain
is
a
network
that
consists
of
spatial
regions,
which
are
functionally
linked.
These
regions
share
information
with
each
other
continually
(16).
Using
the
resting-state
fMRI,
we
can
explore
the
functional
connections
of
the
brain
regions.
Functional
connectivity
of
rs-fMRI
data
is
an
important
issue
with
an
increasing
trend
of
innovations
in
recent
years.
An
important
limitation
of
most
rs-fMRI
studies
in
healthy
adults
is
reliance
on
functional
connectivity
indices
calculated
from
an
entire
scan
session
(17).
In
this
way,
important
information
about
within-scan
temporal
changes
in
functional
connectivity
may
be
lost.
Therefore,
the
present
study
aimed
to
determine
the
functional
connectivity
in
a
single
healthy
human
using
his
repeated
rs-fMRI
data.
The
current
study
reveals
that
whole
brain
network
properties
varied
within
a
single
resting-state
scan
session.
Bharat
et
al
have
associated
the
variations
of
functional
connectivity
with
the
intrinsic
activities
of
resting-state
networks
during
a
single
resting
state
scan
by
comparing
functional
connectivity
differences
between
the
situation
when
a
network
had
higher
and
lower
intrinsic
activities
(18).
Allen
et
al.
have
described
an
approach
to
assess
whole-brain
FC
dynamics
based
on
spatial
independent
component
analysis,
sliding
time
window
correlation,
and
k-means
clustering
of
windowed
correlation
matrices
(19).
There
are
few
good
review
articles
about
dynamic
FC.
Hutchison
et
al
have
reviewed
recent
findings,
methodological
considerations,
neural
and
behavioral
correlates,
and
some
directions
in
the
emerging
field
of
dynamic
FC
studies
(10).
In
addition,
Ioannides
review
FC
results
from
a
variety
of
studies,
which
suggest
that
an
adequate
description
of
brain
organization
requires
a
hierarchy
of
networks
rather
than
a
single
one
(20).
Viviano
et
al
explore
the
associations
between
dynamic
functional
connectivity
and
age
differences,
metabolic
risk,
and
cognitive
performance
in
healthy
adults
(21).
Hutchison
et
al
showed
that
the
Resting-state
networks
have
Dynamic
FC
in
awake
humans
and
anesthetized
macaques.
Their
results
illustrated
that
resting-state
functional
connectivity
is
not
static
(22).
Marusak
et
al
have
explored
the
Dynamic
FC
of
neurocognitive
networks
in
children
in
a
sample
of
146
youth
from
varied
sociodemographic
backgrounds.
They
applied
the
Independent
component
analysis,
sliding
time
window
correlation,
and
k-means
clustering
to
rs-fMRI
data.
Their
results
showed
six
dynamic
FC
networks
that
re-occur
over
time
(23).
Bhattacharya
et
al
have
proposed
a
nonparametric
Bayesian
approach
to
model
effective
connectivity
assuming
a
dynamic
non-stationary
neuronal
system
(24).
However
a
large
number
of
ROIs
is
possible
for
the
variance
model,
but
we
needed
to
make
modifications
to
the
proposed
method
to
accommodate
the
larger
number
of
ROIs.
The
reason
was
that
the
number
of
parameters
in
our
model
were
very
large
compared
with
respect
to
the
number
of
ROIs.
To
solve
this
problem
we
ignored
the
covariance
terms
in
the
between-subject
covariance
matrix.
Because
of
a
small
number
of
sessions,
we
considered
only
the
scaled
identity
structure
for
the
between-subject
covariance
matrix,
since
by
this
structure
the
model
has
a
small
number
of
parameters.
Using
larger
sample
sizes,
one
can
consider
structures
that
are
more
complex.
1.
Zhang
L,
Guindani
M,
Vannucci
M.
Bayesian
models
for
functional
magnetic
resonance
imaging
data
analysis.
Wiley
Interdisciplinary
Reviews:
Computational
Statistics.
2015
Jan
1;7(1):21-41.
2.
Friston
KJ.
Functional
and
effective
connectivity
in
neuroimaging:
a
synthesis.
Hum
Brain
Mapp
1994,
2:5678.
3.
Friston
KJ.
Functional
and
effective
connectivity:
a
review.
Brain
Connectivity
2011,
10:1336.
4.
Zalesky
A,
Fornito
A,
Bullmore
E.
On
the
use
of
correlation
as
a
measure
of
network
connectivity.
Neuroimage
2012,
60:20962106.
5.
Andersen
AH,
Gash
DM,
Avison
MJ.
Principal
component
analysis
of
the
dynamic
response
measured
by
fMRI:
a
generalized
linear
systems
framework.
Magn
Reson
Imaging
1999,
170:795815.
6.
Calhoun
VD,
Adali
T,
Pearlson
GD,
Pekar
JJ.
A
method
for
making
group
inferences
from
functional
MRI
data
using
independent
component
analysis.
Hum
Brain
Mapp
2001,
140:140151.
7.
McKeown
M,
Makeig
S,
Brown
G,
Jung
T,
Kindermann
S,
Bell
A,
Sejnowski
T.
Analysis
of
fMRI
data
by
blind
separation
into
independent
spatial
components.
Hum
Brain
Mapp
1998,
6:160188.
8.
Cribben
I,
Haraldsdottir
R,
Atlas
LY,
Wager
TD,
Lindquist
MA.
Dynamic
connectivity
regression:
determining
state-related
changes
in
brain
connectivity.
Neuroimage
2012,
61:907920.
9.
Varoquaux
G,
Gramfort
A,
Poline
JB,
Thirion
B,
Zemel
R,
Shawe-Taylor
J.
Brain
covariance
selection:
better
individual
functional
connectivity
models
using
population
prior.
In:
Zemel
R,
Shawe-Taylor
J,
eds.
Advances
in
Neural
Information
Processing
System.
10.
Hutchison
RM,
Womelsdorf
T,
Allen
EA,
Bandettini
PA,
Calhoun
VD,
Corbetta
M,
et
al.
Dynamic
functional
connectivity:
Promise,
issues,
and
interpretations.
Neuroimage
[Internet].
Elsevier
Inc.;
2013;80:36078.
Available
from:
http://dx.doi.org/10.1016/j.neuroimage.2013.05.079
11.
Poldrack
RA,
Laumann
TO,
Koyejo
O,
Gregory
B,
Hover
A,
Chen
MY,
Gorgolewski
KJ,
Luci
J,
Joo
SJ,
Boyd
RL,
Hunicke-Smith
S.
Long-term
neural
and
physiological
phenotyping
of
a
single
human.
Nature
communications.
2015
Dec
9;6:8885.
12.
Fiecas
M,
Cribben
I,
Bahktiari
R,
Cummine
J.
A
variance
components
model
for
statistical
inference
on
functional
connectivity
networks.
Neuroimage
[Internet].
Elsevier;
2017;149:25666.
Available
from:
http://dx.doi.org/10.1016/j.neuroimage.2017.01.051
13.
Smith
SM,
Jenkinson
M,
Woolrich
MW,
Beckmann
CF,
Behrens
TE,
Johansen-Berg
H,
Bannister
PR,
De
Luca
M,
Drobnjak
I,
Flitney
DE,
Niazy
RK.
Advances
in
functional
and
structural
MR
image
analysis
and
implementation
as
FSL.
Neuroimage.
2004
Dec
31;23:S208-19.
14.
Jenkinson
M,
Bannister
P,
Brady
M,
Smith
S.
Improved
optimization
for
the
robust
and
accurate
linear
registration
and
motion
correction
of
brain
images.
Neuroimage.
2002
Oct
31;17(2):825-41.
15.
Smith
SM.
Fast
robust
automated
brain
extraction.
Human
brain
mapping.
2002
Nov
1;17(3):143-55.
16.
van
den
Heuvel
MP,
Hulshoff
Pol
HE.
Exploring
the
brain
network:
A
review
on
resting-state
fMRI
functional
connectivity.
Eur
Neuropsychopharmacol
[Internet].
Elsevier
B.V.;
2010;20(8):51934.
Available
from:
http://dx.doi.org/10.1016/j.euroneuro.2010.03.008
17.
Chang
C,
Glover
GH.
Timefrequency
dynamics
of
resting-state
brain
connectivity
measured
with
fMRI.
Neuroimage.
2010
Mar
31;50(1):81-98.
18.
Di
X,
Biswal
BB.
Dynamic
brain
functional
connectivity
modulated
by
resting-state
networks.
Brain
Struct
Funct.
2013;220(1):3746.
19.
Allen
EA,
Damaraju
E,
Plis
SM,
Erhardt
EB,
Eichele
T,
Calhoun
VD.
Tracking
whole-brain
connectivity
dynamics
in
the
resting
state.
Cereb
Cortex.
2014;24(3):66376.
20.
Ioannides
AA.
Dynamic
functional
connectivity.
Curr
Opin
Neurobiol.
2007;17(2):16170.
21.
Viviano
RP,
Raz
N,
Yuan
P,
Damoiseaux
JS.
Associations
between
dynamic
functional
connectivity
and
age,
metabolic
risk,
and
cognitive
performance.
Neurobiol
Aging
[Internet].
Elsevier
Inc.;
2017;
Available
from:
http://linkinghub.elsevier.com/retrieve/pii/S0197458017302580
22.
Hutchison
RM,
Womelsdorf
T,
Gati
JS,
Everling
S,
Menon
RS.
Resting-state
networks
show
dynamic
functional
connectivity
in
awake
humans
and
anesthetized
macaques.
Hum
Brain
Mapp.
2013;34(9):215477.
23.
Marusak
HA,
Calhoun
VD,
Brown
S,
Crespo
LM,
Sala-Hamrick
K,
Gotlib
IH,
et
al.
Dynamic
functional
connectivity
of
neurocognitive
networks
in
children.
Hum
Brain
Mapp.
2017;38(1):97108.
24.
Bhattacharya
S,
Maitra
R.
A
nonstationary
nonparametric
Bayesian
approach
to
dynamically
modeling
effective
connectivity
in
functional
magnetic
resonance
imaging
experiments.
Ann
Appl
Stat.
2011;5(2
B):1183206.
|
|
.................................................................................................................
|
|
|