|
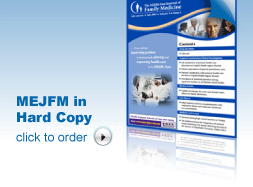 |
............................................................. |
|
........................................................ |
From
the Editor |

|
Editorial
A. Abyad (Chief Editor) |
|
|
........................................................
In Memoriam
Professor
Orhan Ekrem Müftüoglu
|
........................................................
Original
Contribution / Clinical Investigation




|
Cholelithiasis
and cholecystectomy may lower the low density
lipoprotein cholesterol in plasma
DOI: 10.5742/MEWFM.2017.93010
[pdf
version]
Mehmet Rami Helvaci, Mursel Davarci, Orhan Veli
Ozkan, Ersan Semerci, Abdulrazak Abyad, Lesley
Pocock
Serum
and follicular fluid vitamin D and follicular
response among infertile women undergoing ICSI
DOI: 10.5742/MEWFM.2017.93011
[pdf
version]
Sedighe Esmaeilzadeh, Maryam Aliasgharpour,
Parvaneh Mirabi, Azita Ghanbarpour
Maede Fasihian
Studying
the relation of quality of work life with socio-economic
status and general health among the employees
working in Students Welfare Fund of Ministry
of Health and Medical Education in 2016
DOI: 10.5742/MEWFM.2017.93012
[pdf
version]
Saeed Reza Azami, Nasrin Shaarbafchizadeh, Soheil
Mokhtari, Ali Maher
On the Effect
of Cognitive Behavioural Counseling on Sexual
Satisfaction of Mothers with Autistic Children:
A Randomized Clinical Trial
DOI:
[pdf version]
Leila Arbil, Mitra Kolivand, Farzaneh Golboni,
Effat MerghatiKhoei, Mansour Rezaei
Pre-operative
sublingual misoprostol and intra-operative blood
loss during total abdominal hysterectomy: a
randomized single-blinded controlled clinical
trial
DOI: 10.5742/MEWFM.2017.93013
[pdf
version]
Taravat Fakheri, Tayebe Noori
Investigating
the Effect of Endotracheal Tube Cuff Pressure
on Sore Throat, Hoarseness and Cough in Patients
with Coronary Artery Bypass Surgery
DOI: 10.5742/MEWFM.2017.93014
[pdf
version]
Ali Akbar Vaezi, Mohammad Hassan Mondegari Bamakan
Comparing
the Self-Esteem and Resiliency between Blind
and Sighted Children and Adolescents in Kermanshah
City
DOI: 10.5742/MEWFM.2017.93015
[pdf
version]
Saeedeh Bakhshi, Nafiseh Montazeri , Babak Nazari,
Arash Ziapour, Hashem Barahooyi,
Fatemeh Dehghan
|
........................................................
Population
and Community Studies






|
Frequency
of Uric Acid Levels, Symptomatic and Asymptomatic
Hyperuricemia among the Pakistani Population
DOI: 10.5742/MEWFM.2017.93016
[pdf
version]
Waris Qidwai, Masood Jawaid
Determinants
of Tooth Brushing among Primary School Students
DOI: 10.5742/MEWFM.2017.93017
[pdf
version]
Mohammad Mahboubi, Mohammad Ismail Motlagh,
Mehdi Mirzaei-Alavijeh, Farzad Jalilian, Hassan
Gharibnavaz,
Mohammad Fattahi
Depression
in patients suffering from gender dysphoria:
The hospitalized patients of Legal Medicine
Center in Southwest of Iran
DOI: 10.5742/MEWFM.2017.93018
[pdf
version]
Zahra Gorjian, Mohammad Zarenezhad, Mohhamad
Mahboubi, Saeid Gholamzadeh,
Nahid Mahmoodi
An epidemiological
study of suicide attempts and to determine the
correlation between attempted suicide causes
and demographic characteristics of people in
Kermanshah Province during a year
DOI: 10.5742/MEWFM.2017.93019
[pdf
version]
Hamid Reza Shetabi, Samira Rostami, Mohsen Mohammadi,
Mahsa Cheleii, Lida Saedi, Saba Amiri Nasab,
Shirin Zardui GolAnbari
The
effectiveness of life skills training on happiness,
mental health, and marital satisfaction in wives
of Iran-Iraq war veterans
DOI: 10.5742/MEWFM.2017.93038
[pdf
version]
Kamal Solati
The
Role of Self-Compassion Factors in Predicting
the Marital Satisfaction of Staff at Kermanshah
University of Medical Sciences
DOI:10.5742/MEWFM.2017.93020
[pdf
version]
Parisa Janjani, Lida Haghnazari, Farahnaz Keshavarzi,
Alireza Rai
Mediating
role of irrational beliefs in the relationship
between the quality of family communication
and marital satisfaction
DOI:10.5742/MEWFM.2017.93021
[pdf
version]
Parisa Janjani, Khodamorad Momeni, Alireza Rai,
Mohammad Reza Saidi
|
........................................................
Review Article
........................................................
International Health
Affairs
........................................................
Education
and Training
........................................................
Clinical
Research and Methods




|
Adaptive
LASSO Logistic Regression applied on gene expression
of prostate cancer
DOI: 10.5742/MEWFM.2017.93028
[pdf version]
Amir Hossein Hashemian, Maryam Ghobadi Asl,
Soodeh Shahsavari, Mansour Rezaei,
Hadi Raeisi Shahraki
The
prevalence of brain and neck injuries in patients
with maxillofacial fractures in teaching hospitals
of Rasht in 2016
DOI: 10.5742/MEWFM.2017.93029
[pdf
version]
Seyed Mohammad Talebzadeh, Ali Khalighi Sigaroudi,
Babak Alijani, Safa Motevasseli,
Saied Dashtyari, Mahsa Shariati, Zeinab Davoudmanesh
Cultural
competency: a concept analysis in TUMS (Tehran
University of Medical Science) DOI:
10.5742/MEWFM.2017.93030
[pdf version]
Foruzan Khatamidoost, Mandana Shirazy, Hamid
Khankeh, Nemat Allah Musapour
Majid Sadeghi, Kamran Soltani Arabshahi
The
Effect of Proprioceptive Neuromuscular Facilitation
(PNF) on Activities of Daily Living of client
with Cerebrovascular accident
DOI: 10.5742/MEWFM.2017.93031
[pdf
version]
Najafi Doulatabad Shahla, Afrasiabifar Ardashir,
Parandvar Yaghoub
Evaluation
of the ratio of T helper 17 and T regulatory
cells in patients with chronic idiopathic urticaria
DOI: 10.5742/MEWFM.2017.93032
[pdf
version]
Hossein Shahriari, Farahzad Jabbari, Seyyed
Abdolrahim Rezaee, Houshang Rafatpanah
Majid Jafari, Reza Farid Hosseini, Majid Asadi-Samani
|
........................................................
Model
and System of Primary Care
........................................................
Case
Series and Case Reports
Chief
Editor -
Abdulrazak
Abyad
MD, MPH, MBA, AGSF, AFCHSE
.........................................................
Editorial
Office -
Abyad Medical Center & Middle East Longevity
Institute
Azmi Street, Abdo Center,
PO BOX 618
Tripoli, Lebanon
Phone: (961) 6-443684
Fax: (961) 6-443685
Email:
aabyad@cyberia.net.lb
.........................................................
Publisher
-
Lesley
Pocock
medi+WORLD International
11 Colston Avenue,
Sherbrooke 3789
AUSTRALIA
Phone: +61 (3) 9005 9847
Fax: +61 (3) 9012 5857
Email:
lesleypocock@mediworld.com.au
.........................................................
Editorial
Enquiries -
abyad@cyberia.net.lb
.........................................................
Advertising
Enquiries -
lesleypocock@mediworld.com.au
.........................................................
While all
efforts have been made to ensure the accuracy
of the information in this journal, opinions
expressed are those of the authors and do not
necessarily reflect the views of The Publishers,
Editor or the Editorial Board. The publishers,
Editor and Editorial Board cannot be held responsible
for errors or any consequences arising from
the use of information contained in this journal;
or the views and opinions expressed. Publication
of any advertisements does not constitute any
endorsement by the Publishers and Editors of
the product advertised.
The contents
of this journal are copyright. Apart from any
fair dealing for purposes of private study,
research, criticism or review, as permitted
under the Australian Copyright Act, no part
of this program may be reproduced without the
permission of the publisher.
|
|
|
September 2017
- Volume 15, Issue 7 |
|
Adaptive LASSO Logistic
Regression applied on gene expression of prostate
Cancer
Amir Hossein
Hashemian (1,4)
Maryam Ghobadi Asl (2)
Soodeh Shahsavari (3)
Mansour Rezaei (4)
Hadi Raeisi Shahraki (5)
(1) Research Center for Environmental Determinants
of Health (RCEDH), Kermanshah University of
Medical Sciences, Kermanshah, Iran.
(2) Department of Biostatistics and Epidemiology,
School of Public Health, Kermanshah University
of Medical Sciences, Kermanshah, Iran.
(3) Department of Biostatistics and Epidemiology,
School paramedics, Kermanshah University of
Medical Sciences, Kermanshah, Iran.
(4) Department of Biostatistics and Epidemiology,
School of Public Health, Kermanshah University
of Medical Sciences, Kermanshah, Iran.
(5) Department of Biostatistics, School of Medicine,
Shiraz University of Medical Sciences, Shiraz,
Iran
Correspondence:
Maryam Ghobadi Asl
Department of Biostatistics and Epidemiology,
School of Public Health,
Kermanshah University of Medical Sciences,
Kermanshah, Iran
Email: ghobadiasl92@gmail.com
Abstract
Introduction:
The high number of prostate cancer
patients has signified the importance
of identifying its risk factors. The aim
of the present study was to employ stratification
method and penalized logistic regression
with the adaptive LASSO for selecting
appropriate and important genes in prostate
cancer.
Materials and
Methods: Microarray data used in this
study include a prostate cancer gene expression
dataset with [HG_U95B] Affymetrix Human
Genome U95B Array platform which consisted
of 12,620 genes and 167 subjects, among
whom 76 subjects were unaffected and the
rest were affected with cancer. Using
adaptive Lasso regression, important genes
in prostate cancer were stratified and
the results were analyzed using ROC analysis
and gene ontology annotation. To modify
and conduct primary measures on the dataset,
SPSS software version 22 was used. To
fit the models and draw the diagrams,
R software version 3.3.1 and penalty specific
packages were used.
Findings:
According to this research, the obtained
adaptive Lasso regression accuracy and
confidence interval (CI) were 0.99 and
0.97-0.99, respectively. Considering criteria
such as area under the curve and gene
ontology annotation, it can be argued
that adaptive Lasso regression was fairly
effective in stratification and selection
of appropriate genes in prostate cancer.
Conclusion:
Based on the results of this study,
it can be said that in gene expression
data, where there are both linear and
large scale data, techniques such as adaptive
Lasso can be useful in diagnosis of effective
genes.
Key words:
Regression with adaptive LASSO, Prostate
cancer, Gene expression, Stratification,
Gene Ontology annotation.
|
Prostate cancer is the most common and most
dangerous cancer among men, the fifth most common
cancer worldwide, and the second most common
cancer among men(1). In 2012, 1.1 million men
were diagnosed with prostate cancer and 307,000
of them died(2). This cancer is the most common
cancer among men in 84 countries; it mostly
affects developed countries and is rising in
developing countries. Recent incidence rates
of prostate cancer in developed countries and
developing countries are 19% and 5%, respectively(3).
Autopsy studies indicated that about one-third
of over 50 men have microscopic evidence of
prostate cancer; however, most of these cancers
are so slow growing that they never risk the
lives of the affected individuals. Thus, most
of men die of prostate cancer rather than other
types of cancer(4).
Although prostate cancer may occur at any age,
80% of men diagnosed with this type of cancer
are 65 years of age and it rarely occurs in
men under 50 years. Only 2% of prostate cancer
patients are <50 years of age. The average
age of diagnosis of prostate cancer is 68 years
and 63% of cases are diagnosed after 65. About
1 out of 9 men will be diagnosed with prostate
cancer in his lifetime. In recent years, annual
rate of prostate cancer has increased by 4%
in the United States. Prostate cancer is responsible
for 11% of deaths from cancer among American
men(5, 6).
Doctors rarely know why a man develops prostate
cancer and another does not, but statistical
studies show a set of causes for incidence of
prostate cancer in men, the most important of
which are age, genetics, inflammation, infection,
genetic predisposition, dietary factors,
sexually transmitted diseases, lack of vitamin
D, vasectomy, smoking, fat diet, obesity, some
medicines- e.g. daily use of medications such
as anti-inflammatory drugs, prostate specific
changes, and genome-specific changes(6, 7).
Unfortunately, most prostate cancers are asymptomatic
and have no significant symptoms or problems
for months or even years. In general, to identify
warning symptoms for prostate cancer, we should
be familiar with blood in urine, burning while
urinating, slow urinary stream, urinary incontinence,
urinary retention, abdominal pain, general symptoms
of cancer, acute erectile dysfunction, and intense
pain during intercourse. These symptoms may
also be seen in benign enlargement of the prostate.
The main difference between these two is that
in benign enlargement of prostate, symptoms
appear progressively, but prostate cancer may
start quite suddenly(7).
High number of prostate cancer patients has
signified the importance of identifying its
risk factors. With the onset of microarray technology
in 1995, measures were taken to stratify cancers
based on genotypic characteristics(8). Several
studies have shown that only a small subset
of genes have significant association with the
investigated diseases and according to cancer
stratification, many genes are proved to be
non-related. These genes may represent noise
to data and reduce stratification accuracy.
In addition, these genes may cause more fitting
to model and leave adverse effects on stratifications
and due to the presence of the importance of
such issues, it was necessary to introduce methods
for effective gene selection given the situation
and improve the accuracy of prediction. Several
statistical methods have been introduced for
cancer stratification among which logistic regression
is regarded as a powerful method for gene differentiation;
however, this method is neither appropriate
nor applicable for stratification of large scale
data and thus repetitive methods such as Newton-Raphson
cannot be applied. Recently, penalized methods
have been used for stratification of large-scale
cancer data. In order to estimate coefficients
of genes and select appropriate genes, Penalized
Logistic Regression has been successful in stratification
of large-scale cancer data(9). Therefore, the
current research was conducted to employ effective
stratification method and Adaptive LASSO Logistic
Prognosis for selecting appropriate and important
genes in prostate cancer.
This
is
a
cross-sectional
study
aimed
at
measuring
the
application
of
logistic
regression
using
Adaptive
LASSO
in
studying
the
association
between
different
genes
and
prostate
cancer.
Required
information
were
downloaded
from
ncbi.nlm.nih.gov
website,
GEO
gene
expression
data
sets.
Here,
a
prostate
cancer
gene
expression
dataset
with
[HG_U95B]
Affymetrix
Human
Genome
U95B
Array
platform
was
used.
A
total
of
167
subjects
participated
among
whom
76
subjects
were
unaffected
and
the
rest
were
affected.
Also,
12,620
genes
were
analyzed.
Thus,
the
prostate
cancer
gene
expression
dataset
dimension
was
12,620
x
167.
Since
data
obtained
from
the
matrix
of
microarray
technology
for
prostate
cancer
data
are
raw
data
and
require
pre-processing
to
be
used,
various
statistical
methods,
called
normalization,
were
performed
to
remove
or
minimize
some
of
the
unwanted
changes
brought
about
by
biases
and
laboratory
or
technical
inconsistencies.
Then,
they
were
imputed
for
missing
values
and
filtering
methods
were
used
to
remove
large-scale
data
effects.
Quantiles
were
used
in
this
method;
values
less
than
the
first
quarter
and
larger
than
the
third
quarter
were
excluded.
Using
filtering
method,
the
number
of
examined
genes
was
reduced
to
3,145.
To
select
the
most
important
and
significant
features
used
in
stratification,
the
next
step
was
to
select
important
variables
which
were
allowed
to
participate
in
stratification.
In
order
to
be
able
to
identify
significant
genes,
data
scale
should
be
reduced.
PLS
method
was
used
for
reducing
data
dimensions.
PLS
model
was
conducted
because
of
having
large
scale
and
correlated
data.
PLS-DA
regression
was
used
for
gene
expression.
Adaptive
LASSO
method
was
employed
for
stratification
of
important
genes
in
prostate
cancer.
To
modify
and
conduct
primary
measures
on
the
dataset,
SPSS
software
version
22
was
used.
To
fit
the
models
and
draw
the
diagrams
R
software
version
3.3.1
and
penalty
specific
packages
were
used.
Finally,
the
results
were
analyzed
using
ROC
analysis
and
gene
ontology
annotation.
PLS-DA
model
fitting
for
selecting
important
variables
in
stratification
analysis
After
normalization,
the
goal
was
to
select
important
variables
in
stratification.
Thus,
according
to
PLS-DA
model,
variables
with
p-value
<0.01
were
included
(n=1700)
(p-value
calculation
for
Variable
Importance
in
the
Projection
index).
Figure
1
shows
variables
significance
based
on
their
P-values.
Click
here
for
Figure
1:
The
importance
of
studied
genes
using
PLS-DA
model
fitting
The
Application
of
Lasso
Adaptive
Regression
Model
for
the
studied
data
Regularization
Parameter
Estimation
To
find
the
best
regularization
parameter,
47
different
modes
are
regulated
for
47
Lasso
adaptive
regressions
with
estimated
regularization
parameter
so
as
to
find
the
best
for
the
best
value
(Figure
2).
Click
here
for
Figure
2:
Regularization
parameter
estimation
in
47
different
modes
Then,
adaptive
Lasso
regression
model
with
estimated
regularization
parameter
was
carried
out
on
the
study
dataset
based
on
which
the
variables
were
stratified.
Accuracy
of
area
under
the
ROC
curve
was
0.99
(95%
CI:0.97-0.99)
(Figure
3).
Click
here
for
Figure
3:
ROC
curve
obtained
from
stratification
based
on
adaptive
Lasso
regression
model
with
estimated
regularization
parameter
Biovalidation
of
data
from
stratification
of
prostate
cancer
gene
expression
data
In
order
to
properly
measure
predicted
models
for
stratification
of
prostrate
cancer
gene
expression
data
using
Gene
Ontology
(GO),
it
was
determined
that,
based
on
a
specific
GO
interpretation
in
different
models,
whether
the
set
of
genes
obtained
from
stratification
are
significant
or
not.
The
most
significant
GO
terms
used
to
describe
the
identified
genes
at
P-value<0.05
are
shown.
Stratification
rate
of
the
studied
models
were
between
76
to
89%
(Table
1).
Table
1:
GO
analysis
of
prostate
cancer
gene
expression
data
Medical
studies
in
recent
years,
and
especially
after
the
publication
of
information
about
sequencing
the
human
genome
project,
have
experienced
a
new
field
that
opens
promising
ways
for
researchers
to
identify
and
treat
incurable
diseases,
such
as
cancer.
Yet,
unlike
medical
data
collected
in
earlier
studies
where
researchers
have
been
dealing
with
a
small
number
of
variables,
the
new
field
of
cellular
and
molecular
Medicine
is
faced
with
a
very
high
volume
of
information
which
is
being
produced
by
laboratory-based
methods
and
techniques.
One
of
the
most
efficient
methods
to
extract
genetic
information
is
microarray
method
which
has
given
researchers
very
high
potential
for
extracting
huge
volumes
of
information
instantaneously
and
according
to
arbitrary
conditions.
It
is
one
of
the
most
controversial
scientific
topics
in
the
field
of
computational
methods,
management
methods,
and
analysis
of
this
type
of
data.
Given
the
researchers
goal
of
using
microarray
techniques
in
data
mining,
which
is
understanding
the
function
of
genes
in
cell
activities,
computational
methods
related
to
this
area,
known
as
stratification
methods,
are
also
more
important
and
one
of
the
most
important
questions
which
have
been
raised
on
the
use
of
these
methods
was
to
identify
the
method
or
methods
with
proper
function
of
gene
stratification.
Adaptive
Lasso
regression
was
evaluated
in
different
conditions.
The
best
value
estimated
for
regularization
parameter
in
47
different
modes
was
3.79,
with
99%
accuracy
and
CI
between
97%-99%
for
area
under
the
ROC
curve
which
was
a
very
suitable
model
with
more
acceptable
performance
than
other
values
estimated
for
regularization
parameter.
Tibshirani
investigated
the
effect
of
8
variables
on
prostate
specific
antigen
using
data
from
the
prostate
cancer
in
analysis
of
Lasso
model
performance
and
its
comparison
with
least
squares
method
and
the
best
subset.
Results
showed
equality
of
the
best
subset
and
Lasso
and
both
introduced
3
variables
as
influential
ones(10).
He
also
used
penalized
model,
for
the
first
time
in
1997,
for
survival
analysis
and
variable
selection
in
Cox
model.
Using
Lasso
method,
he
studied
the
effect
of
6
variables
on
survival
time
of
patients
with
lung
cancer(11).
Li
and
Fan
(2001)
introduced
3
features
for
a
good
penalty
model,
i.e.
unbiasedness,
sparsity
and
continuity.
As
Lasso
model
may
lead
to
over-estimation
with
big
penalty
coefficient,
it
is
not
unbiased
in
all
conditions.
Techniques,
such
as
ridge
regression,
lack
sparsity
features,
as
well,
because
it
has
not
the
potential
of
excluding
insignificant
variables
from
the
model.
Also,
criteria
such
as
Akaike
criterion
(for
variable
selection)
or
the
best
subset
method
lack
the
third
feature
because
of
instability
in
variable
selection.
Yet,
given
these
3
features,
Fan
proposed
a
penalty
function
called
Smoothly
Clipped
Absolute
Deviation
(SCAD).
The
major
difference
between
SCAD
and
Lasso
is
that
the
former
considers
fixed
penalties
for
coefficients
bigger
than
a.
Considering
7.3
as
an
optimum
value
for
a
resulted
in
SCAD
method
notation
solely
with
subscript(12).
Efron
et
al.
(2004)
introduced
least
angle
regression.
Inspired
by
forward
selection,
in
each
step
of
this
method,
only
one
variable
can
be
entered.
The
advantage
of
Least
Angle
Regression
(LARS)
is
that,
with
a
simple
modification
and
no
need
for
complex
mathematical
algorithms,
all
estimates
may
be
calculated
using
Lasso(13).
Zou
and
Hastie
(2005)
used
elastic
net
and
blood
cancer
data
to
identify
genetic
factors
affecting
cancer
and
predict
its
types
(I
or
II)
by
these
genes.
They
used
blood
cancer
data
of
7,129
genes
selected
by
t1000
gene
statistics
which
had
the
highest
significance
level.
Sample
sizes
of
training
data
and
test
were
38
and
34,
respectively(14).
Huang
et
al.
(2008)
compared
Lasso
and
iterative
Lasso
(adaptive
Lasso
with
reverse
weight
of
Lasso
coefficients)
using
breast
cancer
data.
At
first,
they
selected
500
genes
with
the
largest
absolute
values
of
correlation
coefficients
and
obtained
missed
values
using
median
values.
Then,
Leave-one-out
cross
validation
(LOOCV)
method
was
used
to
calculate
prediction
error.
The
obtained
results
suggested
more
sparsity
of
the
model
proposed
by
iterative
Lasso
so
that
it
selected
only
22
genes
while
Lasso
selected
42
genes
with
the
same
function(15).
Raeisi
Shahraki
et
al.
(2016)
used
Lasso
and
adaptive
Lasso
to
identify
genes
affecting
bladder
cancer.
In
this
study,
with
sample
size
of
48,
expression
of
22
different
genes
in
peripheral
blood
of
people
with
bladder
cancer
was
compared
with
control
group
using
logistic
regression,
Lasso,
and
adaptive
Lasso
so
as
to
identify
genes
which
can
increase
or
decrease
the
risk
of
bladder
cancer.
The
first
notable
point
was
that
Lasso
and
adaptive
Lasso
methods
could
be
fitted
to
data,
despite
high
correlation
between
some
variables,
by
considering
all
22
variables;
however,
logistic
regression
was
not
able
to
converge
even
by
taking
one
third
of
variables
into
account.
By
controlling
the
multicollinearity,
adaptive
Lasso
method
was
well
fitted
to
data
and
estimated
coefficients
with
a
very
high
accuracy
and
low
error.
The
model
proposed
by
this
method
was
a
reliable
model
with
many
other
optimal
features
such
as
reliability,
compatibility,
predictability,
sparsity,
and
ideal
flexibility(16).
Due
to
massive
amounts
of
gene
expression
data,
which
is
an
important
characteristic
of
this
study,
results
obtained
from
various
studies
showed
that
all
the
previous
research
has
been
conducted
on
a
limited
number
of
genes.
Since
1996,
introduction
of
microarray
technology
in
simultaneous
expression
of
thousands
of
genes
revolutionized
analysis
of
genes
so
as
not
to
limit
gene
analysis
to
few
number
of
genes.
Considering
that
microarray
knowledge
is
used
in
this
study,
various
models
are
predicted
and
compared,
final
results
are
evaluated
using
ROC
analysis
and
validation
of
gene
ontology,
and
the
most
appropriate
stratification
method
is
carried
out
to
identify
significant
genes,
it
is
not
so
much
similar
to
previous
studies.
Based
on
the
results
of
this
study,
in
gene
expression
data,
where
there
are
both
linear
and
large
scale
data,
techniques
such
as
adaptive
Lasso
provide
higher
performance
in
stratification
aid
diagnosis
of
effective
genes.
Acknowledgment:
This
research
is
derived
from
a
thesis
approved
by
deputy
of
research
at
Kermanshah
University
of
Medical
Science
numbered
95072.
1.
Grubb
3rd
R,
Kibel
A.
Prostate
cancer:
screening,
diagnosis
and
management
in
2007.
Missouri
medicine.
2006;104(5):408-13;
quiz
13-4.
2.
Organization
WH.
Global
status
report
on
noncommunicable
diseases
2014:
World
Health
Organization;
2014.
3.
Parkin
DM,
Bray
F,
Ferlay
J,
Pisani
P.
Global
cancer
statistics,
2002.
CA:
a
cancer
journal
for
clinicians.
2005;55(2):74-108.
4.
Torre
LA,
Bray
F,
Siegel
RL,
Ferlay
J,
LortetTieulent
J,
Jemal
A.
Global
cancer
statistics,
2012.
CA:
a
cancer
journal
for
clinicians.
2015;65(2):87-108.
5.
Ross
DT,
Scherf
U,
Eisen
MB,
Perou
CM,
Rees
C,
Spellman
P,
et
al.
Systematic
variation
in
gene
expression
patterns
in
human
cancer
cell
lines.
Nature
genetics.
2000;24(3):227-35.
6.
Ruijter
E,
van
de
Kaa
C,
Miller
G,
Ruiter
D,
Debruyne
F,
Schalken
J.
Molecular
genetics
and
epidemiology
of
prostate
carcinoma.
Endocrine
reviews.
1999;20(1):22-45.
7.
Carter
H.
partin
AW.
Diagnosis
and
staging
of
prostate
cancer.
Campbell-Walsh
Urology
8th
ed
Sydney:
Elsevier
Health
Sciences.
2002.
8.
Meyer
C,
Davis
S.
Its
alive:
The
coming
convergence
of
information,
biology,
and
business:
Crown
Business;
2003.
9.
Carter
HB,
Partin
AW.
Diagnosis
and
staging
of
prostate
cancer.
Campbells
urology.
2002;3:2519-37.
10.
Tibshirani
R.
Regression
shrinkage
and
selection
via
the
lasso.
Journal
of
the
Royal
Statistical
Society
Series
B
(Methodological).
1996:267-88.
11.
Tibshirani
R.
The
lasso
method
for
variable
selection
in
the
Cox
model.
Statistics
in
medicine.
1997;16(4):385-95.
12.
Fan
J,
Li
R.
Variable
selection
via
nonconcave
penalized
likelihood
and
its
oracle
properties.
Journal
of
the
American
statistical
Association.
2001;96(456):1348-60.
13.
Efron
B,
Hastie
T,
Johnstone
I,
Tibshirani
R.
Least
angle
regression.
The
Annals
of
statistics.
2004;32(2):407-99.
14.
Zou
H,
Hastie
T.
Regularization
and
variable
selection
via
the
elastic
net.
Journal
of
the
Royal
Statistical
Society:
Series
B
(Statistical
Methodology).
2005;67(2):301-20.
15.
Huang
J,
Ma
S,
Zhang
C-H.
The
iterated
lasso
for
high-dimensional
logistic
regression.
The
University
of
Iowa,
Department
of
Statistics
and
Actuarial
Sciences.
2008.
16.
Shahraki
HR,
Jaberipoor
M,
Zare
N,
Hosseini
A.
The
Role
of
22
Genes
Expression
in
Bladder
Cancer
by
Adaptive
LASSO.
2016.
|
|
.................................................................................................................
|
|
|